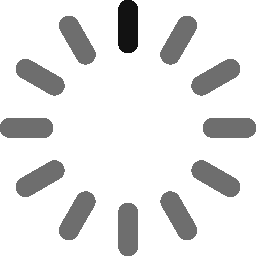
Related items loading ...
Section 1: Publication
Publication Type
Journal Article
Authorship
Harder, P., Pomeroy, J., & Helgason, W.
Title
Improving sub-canopy snow depth mapping with unmanned aerial vehicles: lidar versus structure-from-motion techniques
Year
2020
Publication Outlet
The Cryosphere, 14(6): 1919-1935
DOI
ISBN
ISSN
ISSN 0048-9697
Citation
Harder, P., Pomeroy, J., & Helgason, W. (2020). Improving sub-canopy snow depth mapping with unmanned aerial vehicles: lidar versus structure-from-motion techniques. The Cryosphere, 14(6): 1919-1935.
https://doi.org/10.5194/tc-14-1919-2020
Abstract
Vegetation has a tremendous influence on snow processes and snowpack dynamics, yet remote sensing techniques to resolve the spatial variability of sub-canopy snow depth are not always available and are difficult from space-based platforms. Unmanned aerial vehicles (UAVs) have had recent widespread application to capture high-resolution information on snow processes and are herein applied to the sub-canopy snow depth challenge. Previous demonstrations of snow depth mapping with UAV structure from motion (SfM) and airborne lidar have focussed on non-vegetated surfaces or reported large errors in the presence of vegetation. In contrast, UAV-lidar systems have high-density point clouds and measure returns from a wide range of scan angles, increasing the likelihood of successfully sensing the sub-canopy snow depth. The effectiveness of UAV lidar and UAV SfM in mapping snow depth in both open and forested terrain was tested in a 2019 field campaign at the Canadian Rockies Hydrological Observatory, Alberta, and at Canadian prairie sites near Saskatoon, Saskatchewan, Canada. Only UAV lidar could successfully measure the sub-canopy snow surface with reliable sub-canopy point coverage and consistent error metrics (root mean square error (RMSE) <0.17 m and bias −0.03 to −0.13 m). Relative to UAV lidar, UAV SfM did not consistently sense the sub-canopy snow surface, the interpolation needed to account for point cloud gaps introduced interpolation artefacts, and error metrics demonstrated relatively large variability (RMSE<0.33 m and bias 0.08 to −0.14 m). With the demonstration of sub-canopy snow depth mapping capabilities, a number of early applications are presented to showcase the ability of UAV lidar to effectively quantify the many multiscale snow processes defining snowpack dynamics in mountain and prairie environments.
Plain Language Summary
Highlights
• A novel wastewater-based risk index simplified understanding of viral load
• Significant correlation relationship between wastewater RNA loads and clinical data
• Thresholds derived from daily per capita viral loads and clinic Rt estimates
• Wastewater-based risk index valuable for decision-making of COVID-19 risk
Section 2: Additional Information
Program Affiliations
Project Affiliations
Submitters
Publication Stage
Published
Theme
Water Quality and Aquatic Ecosystems
Presentation Format
10-minute oral presentation
Additional Information
TTSW