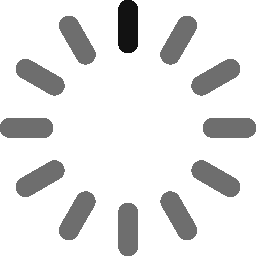
Related items loading ...
Section 1: Publication
Publication Type
Conference Poster
Authorship
Burke, S.A., Palace, M.W., Sullivan, F., et al.
Title
Beyond what the eye can see: using high-resolution UAS-derived hyperspectral imagery to classify boreal peatland vegetation
Year
2022
Publication Outlet
American Geophysical Meeting, Chicago, United States of America, (December 12-16)
DOI
ISBN
ISSN
Citation
Burke, S.A., Palace, M.W., Sullivan, F., et al. (2022) Beyond what the eye can see: using high-resolution UAS-derived hyperspectral imagery to classify boreal peatland vegetation. American Geophysical Meeting, Chicago, United States of America, (December 12-16).
https://agu.confex.com/agu/fm22/meetingapp.cgi/Paper/1148519
Abstract
In Arctic-boreal regions, climate change induced permafrost thaw causes complex interactions between topography, microbiology, hydrology and vegetation distribution that impact the emission rate of radiatively important gasses like methane (CH4). Vegetation distribution, for example, can be used as a proxy for permafrost thaw and the greenhouse gas emissions associated with each stage. To scale emissions up from the site level, more accurate remotely sensed data is needed to produce the necessary vegetation cover maps. Analyses of data collected from unpiloted aerial systems (UAS) have proven effective at classifying vegetation distribution into land cover classes that often include multiple species. However, focusing classification at the level of species functional group (SFG) could further improve scaling efforts by representing vegetation distribution, and thus permafrost thaw impacts and gas emissions, more accurately. High resolution, UAS-derived, hyperspectral imagery was collected in tandem with vegetation surveys, porewater CH4 concentration and isotopes as well as chamber flux measurements to predict six SFG at two boreal peatland sites: Lutose, Alberta (59.5°N 117.2°W) and Smith Creek, NWT (63.2°N 123.3°W). Using a Random Forest Model, we classified the imagery into predetermined land cover classes representative of a permafrost thaw gradient (Permafrost Peat Plateau, Plateau Edge, Young Bog, Intermediate Bog, Mature/Old Bog, and Fen). Each class was spectrally distinct from one another. We then used an Artificial Neural Network to predict the distribution of six SFGs, chosen based on their association with higher or lower CH4 emissions (Carex/Sedge, Scheuchzeria spp., Sphagnum spp., Black Spruce, Lichens, and Cloudberry). Five spectral indices, indicative of structural or chemical properties of vegetation, were used to predict each SFG. We determined which two indices best predicted the distribution of each functional group using a jackknife validation approach. We found we could confidently predict five of the six SFGs, and could produce distribution maps of each SFG in both sites. These data could then be used to parameterize ecosystem models used to predict and then scale CH4 emissions at the site and regional scale
Plain Language Summary