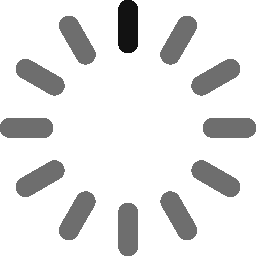
Related items loading ...
Section 1: Publication
Publication Type
Journal Article
Authorship
Razavi, S., Hannah, D. M., Elshorbagy, A., Kumar, S., Marshall, L., Solomatine, D. P., Dezfuli, A., Sadegh, M., Famiglietti, J.
Title
Coevolution of machine learning and process-based modelling to revolutionize Earth and environmental sciences: A perspective
Year
2022
Publication Outlet
Hydrological Processes, 36(6), e14596
DOI
ISBN
ISSN
Citation
Razavi, S., Hannah, D. M., Elshorbagy, A., Kumar, S., Marshall, L., Solomatine, D. P., Dezfuli, A., Sadegh, M., Famiglietti, J. (2022) Coevolution of machine learning and process-based modelling to revolutionize Earth and environmental sciences: A perspective. Hydrological Processes, 36(6), e14596.
https://doi.org/10.1002/hyp.14596
Abstract
Machine learning (ML) applications in Earth and environmental sciences (EES) have gained incredible momentum in recent years. However, these ML applications have largely evolved in ‘isolation’ from the mechanistic, process-based modelling (PBM) paradigms, which have historically been the cornerstone of scientific discovery and policy support. In this perspective, we assert that the cultural barriers between the ML and PBM communities limit the potential of ML, and even its ‘hybridization’ with PBM, for EES applications. Fundamental, but often ignored, differences between ML and PBM are discussed as well as their strengths and weaknesses in light of three overarching modelling objectives in EES, (1) nowcasting and prediction, (2) scenario analysis, and (3) diagnostic learning. The paper ponders over a ‘coevolutionary’ approach to model building, shifting away from a borrowing to a co-creation culture, to develop a generation of models that leverage the unique strengths of ML such as scalability to big data and high-dimensional mapping, while remaining faithful to process-based knowledge base and principles of model explainability and interpretability, and therefore, falsifiability
Plain Language Summary