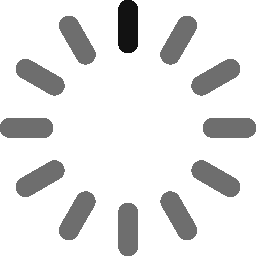
Related items loading ...
Section 1: Publication
Publication Type
Conference Poster
Authorship
Chegoonian, A.M., Leavitt, P.R., Zolfaghari, K., Davies, J.-M., Baulch, H.M., Duguay, C.R.
Title
A regional approach for chlorophyll-a retrieval from small eutrophic lakes via Sentinel-2
Year
2022
Publication Outlet
2022 IEEE International Geoscience & Remote Sensing Symposium, Kuala Lumpur, Malaysia, 17-22 July
DOI
ISBN
ISSN
Citation
Chegoonian, A.M., Leavitt, P.R., Zolfaghari, K., Davies, J.-M., Baulch, H.M., Duguay, C.R. (2022) A regional approach for chlorophyll-a retrieval from small eutrophic lakes via Sentinel-2. 2022 IEEE International Geoscience & Remote Sensing Symposium, Kuala Lumpur, Malaysia, 17-22 July.
https://doi.org/10.1109/IGARSS46834.2022.9883793
Abstract
Remote retrieval of near-surface chlorophyll-a (Chla) concentration from small inland waters adopts two different approaches: development of local models using in situ Chla measurements in target waterbodies or application of globally trained models with no need of local in situ measurements. While the abundance and diversity of small lakes undermine the former approach, application of global models often biases estimations due to uncertainties of atmospheric correction. Here, we introduce a regional approach wherein local in situ data from select waterbodies is used to retrieve Chla in nearby lakes. We trained semi-empirical (SE) and machine-learning (ML) algorithms, namely 2band, LMDN, and SVR, over four ice-free seasons (2017–2020) using 193 pairs of near-surface Chla in Buffalo Pound Lake, Canada, and near-coincident satellite-derived remote-sensing reflectance from Sentinel-2. Ability of locally trained models and a globally trained mixture density network (MDN) to retrieve Chla for unseen data (N=50) was evaluated for six other catchment lakes. Regional approaches based on locally trained ML models (SVR, LMDN) unbiasedly estimated Chla in test sites (overall error ?60% ) and outperformed locally trained SE models and globally trained ML models (reduced error by 20%, bias by 50%). These findings suggest that ML-based regional models are capable of expanding regional Chla retrieval from optically-similar waterbodies
Plain Language Summary