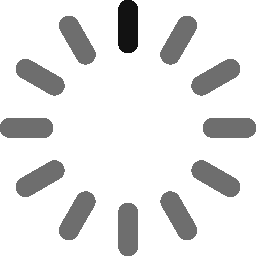
Related items loading ...
Section 1: Publication
Publication Type
Journal Article
Authorship
Shahvaran, A.R., Kheyrollah Pour, H., Van Cappellen, P.
Title
Comparative evaluation of semi-empirical approaches to retrieve satellite-derived chlorophyll-a concentrations from nearshore and offshore waters of a large lake (Lake Ontario)
Year
2024
Publication Outlet
Remote Sensing
DOI
ISBN
ISSN
Citation
Shahvaran, A.R., Kheyrollah Pour, H., Van Cappellen, P. (2024) Comparative evaluation of semi-empirical approaches to retrieve satellite-derived chlorophyll-a concentrations from nearshore and offshore waters of a large lake (Lake Ontario), Remote Sensing,
https://doi.org/10.3390/rs16091595
Abstract
Chlorophyll-a concentration (Chl-a) is commonly used as a proxy for phytoplankton abundance in surface waters of large lakes. Mapping spatial and temporal Chl-a distributions derived from multispectral satellite data is therefore increasingly popular for monitoring trends in trophic state of these important ecosystems. We evaluated products of eleven atmospheric correction processors (LEDAPS, LaSRC, Sen2Cor, ACOLITE, ATCOR, C2RCC, DOS 1, FLAASH, iCOR, Polymer, and QUAC) and 27 reflectance indexes (including band-ratio, three-band, and four-band algorithms) recommended for Chl-a concentration retrieval. These were applied to the western basin of Lake Ontario by pairing 236 satellite scenes from Landsat 5, 7, 8, and Sentinel-2 acquired between 2000 and 2022 to 600 near-synchronous and co-located in situ-measured Chl-a concentrations. The in situ data were categorized based on location, seasonality, and Carlson’s Trophic State Index (TSI). Linear regression Chl-a models were calibrated for each processing scheme plus data category. The models were compared using a range of performance metrics. Categorization of data based on trophic state yielded improved outcomes. Furthermore, Sentinel-2 and Landsat 8 data provided the best results, while Landsat 5 and 7 underperformed. A total of 28 Chl-a models were developed across the different data categorization schemes, with RMSEs ranging from 1.1 to 14.1 ?g/L. ACOLITE-corrected images paired with the blue-to-green band ratio emerged as the generally best performing scheme. However, model performance was dependent on the data filtration practices and varied between satellites.
Plain Language Summary