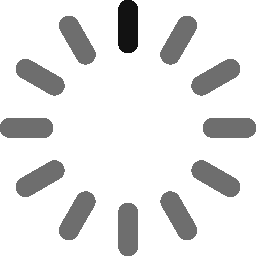
Related items loading ...
Section 1: Publication
Publication Type
Thesis
Authorship
Oladipo, Akintunde
Title
Scaling pre-training data & language models for african languages
Year
2024
Publication Outlet
UWSpace - Theses
DOI
ISBN
ISSN
Citation
Abstract
Recent advancements in language models, particularly for high-resource languages, have not been paralleled in low-resource languages spoken across Africa. This thesis addresses this gap by scaling pre-training data and developing improved language models for African languages. We introduce Wura, a high-quality, document-level pre-training dataset encompassing 16 African languages along with four high-resource languages commonly spoken on the continent: Arabic, English, French, and Portuguese. Leveraging Wura, we pre-train new versions of the AfriBERTa (encoder-only) and AfriTeVa (encoder-decoder) model families. These new models demonstrate superior performance across a variety of natural language understanding and generation tasks compared to existing baselines. Notably, AfriTeVa V2 Large (1B) stands as the largest sequence-to-sequence model pre-trained for African languages to date. Our methodology includes a meticulous three-stage curation process for Wura --- auditing and filtering existing web crawls, initiating new web crawls, and integrating existing language resources. The experimental setup and evaluation encompass tasks like text classification, information retrieval, translation, summarization, and cross-lingual question answering. Our new models outperform their predecessors and other established models, even those with significantly more parameters, highlighting the efficacy of high-quality pre-training data. Furthermore, we study the generalization of our models to languages not deliberately included in their pre-training data.
Plain Language Summary