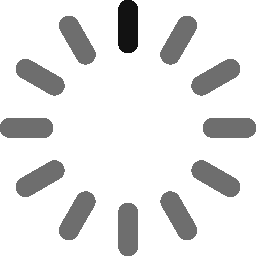
Related items loading ...
Section 1: Publication
Publication Type
Thesis
Authorship
Yee, Lauren
Title
Spatial Modelling and Wildlife Health Surveillance: A case study of White Nose Syndrome in Ontario
Year
2018
Publication Outlet
Scholars Commons Laurier - Theses and Dissertations
DOI
ISBN
ISSN
Citation
Yee, Lauren (2018) Spatial Modelling and Wildlife Health Surveillance: A case study of White Nose Syndrome in Ontario, Scholars Commons Laurier - Theses and Dissertations,
https://scholars.wlu.ca/etd/2040
Abstract
Wildlife data is often limited by survey effort, small sample sizes, and spatial biases associated with collection and missing data. These factors can create unique challenges from a surveillance perspective when trying to extract spatial patterns of habitat suitability and disease distributions for conservation and management purposes. This thesis examined data quality from a wildlife health database in the context of spatial analysis of wildlife disease. Spatial analysis of the data to predict habitat suitability of bats and white nose syndrome afflicted bats was examined by using the MaxEnt modelling method. Methods to reduce spatial bias were examined and specific settings and parameters were evaluated and compared to the default settings in MaxEnt. This analysis showed that subsampling of occurrences leads to less informative models and increased similarity to accessible locations, whereas restricting background locations increased model performance and the resulting suitability maps showed new suitable areas not present in models with default settings. Alternative data sources of bat occurrence data were used from the Global Biodiversity Information Facility which showed distributions were more related to climatic covariates than CWHC models, which were heavily reliant on hibernacula locations. This suggests that CWHC records may benefit from supplementation of publicly available occurrence data. This thesis provides a basis for data quality analyses that should be performed on surveillance data before undertaking more complex modelling procedures.
Plain Language Summary