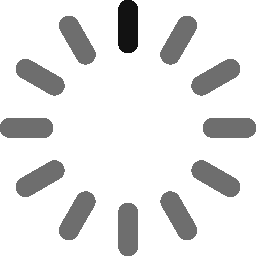
Related items loading ...
Section 1: Publication
Publication Type
Thesis
Authorship
Zhou, Pengxiao
Title
Development of data-driven approaches for wastewater modeling
Year
2023
Publication Outlet
MacSphere Open Access Dissertations and Theses
DOI
ISBN
ISSN
Citation
Zhou, Pengxiao (2023) Development of data-driven approaches for wastewater modeling, MacSphere Open Access Dissertations and Theses,
http://hdl.handle.net/11375/28439
Abstract
To effectively operate and manage the complex wastewater treatment system, simplified representations, known as wastewater modeling, are critical. Wastewater modeling allows for the understanding, monitoring, and prediction of wastewater treatment processes by capturing intricate relationships within the system. Process-driven models (PDMs), which rely on a set of interconnected hypotheses and assumptions, are commonly used to capture the physical, chemical, and biological mechanisms of wastewater treatment. More recently, with the development of advanced algorithms and sensor techniques, data-driven models (DDMs) that are based on analyzing the data about a system, specifically finding relationships between the system state variables without relying on explicit knowledge of the system, have emerged as a complementary alternative. However, both PDMs and DDMs suffer from their limitations. For example, uncertainties of PDMs can arise from imprecise calibration of empirical parameters and natural process variability. Applications of DDMs are limited to certain objectives because of a lack of high-quality dataset and struggling to capture changing relationship. Therefore, this dissertation aims to enhance the stable operation and effective management of WWTPs by addressing these limitations through the pursuit of three objectives: (1) investigating an efficient data-driven approach for uncertainty analysis of process-driven secondary settling tank models; (2) developing data-driven models that can leverage sparse and imbalanced data for the prediction of emerging contaminant removal; (3) exploring an advanced data-driven model for influent flow rate predictions during the COVID-19 emergency.
Plain Language Summary