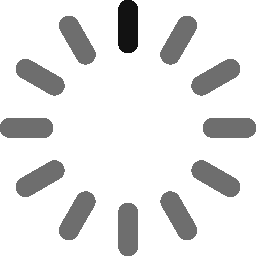
Related items loading ...
Section 1: Publication
Publication Type
Thesis
Authorship
Akbarpour, Shaghayegh
Title
Using Machine Learning to Understand the Hydrologic Impacts of Permafrost Thaw-Driven Land Cover Change
Year
2023
Publication Outlet
UWSpace - Theses
DOI
ISBN
ISSN
Citation
Akbarpour, Shaghayegh (2023) Using Machine Learning to Understand the Hydrologic Impacts of Permafrost Thaw-Driven Land Cover Change, UWSpace - Theses,
http://hdl.handle.net/10012/19322
Abstract
Discontinuous permafrost regions are experiencing a change in land cover distribution as a result of permafrost thaw. In wetlands interspersed with discontinuous permafrost, climate change is particularly problematic because temperature increases can result in significant permafrost thaw, thaw-driven landscape changes, and resultant changes in watershed hydrologic responses. The influence of land cover change on the short- and long-term hydrological responses of wetland-peatland complexes is poorly understood. A better understanding of the impacts of climate-related land cover evolution on the hydrology of wetland-covered watersheds requires information about the distribution of hydrologically important lands, their pattern, and the rate at which they change over time. Here, we first developed a machine learning-based land cover evolution model (TSLCM) to estimate the long-term evolution of dominant land covers for application to the discontinuous permafrost regions of Northern Canada. This model is applied to replicate historical land cover and estimate future land cover scenarios at the Scotty Creek Research Basin in the Northwest Territories, Canada. A significant challenge when analyzing land cover change effects on hydrological properties is generating time-dependent classified maps of the region of interest, and the challenges associated with preprocessing remotely sensed data for discriminating between wetlands and forest-covered regions. In this work, we focus on two important objectives supporting the improved classification of wetlands in discontinuous permafrost regions: the exclusive use of only RGB imagery, and the use of an image segmentation method to accelerate the automatic classification of land cover. A semantic segmentation neural network, a multi-layer perceptron (MLP), and watershed function algorithms are applied to develop the taiga wetland identification neural network (TWINN) for the hydrological classification of wetlands. TWINN is here demonstrated to accurately classify high-resolution imagery of discontinuous permafrost regions within the Northwest Territories into the water, forest, and wetlands, and also able to delineate the runoff area of wetlands. To study the effect of land cover evolution on runoff generation in the Scotty Creek basin, the products of TWINN and TSLCM are used to inform a process-based hydrological model where land cover change is represented explicitly. According to simulation results, land cover transitions can modify annual mean streamflow by as much as 7%, in addition to influences due to changing precipitation regimes alone.
Plain Language Summary