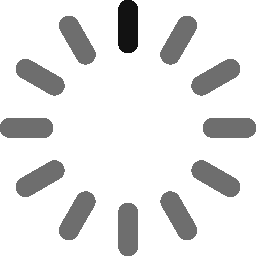
Related items loading ...
Section 1: Publication
Publication Type
Journal Article
Authorship
Al-Ani, Sama; Guo, Hui; Fyfe, Sheila; Long, Zebo; Donnaz, Sylvain; Kim, Younggy
Title
Deep learning-based image analysis for filamentous and floc-forming bacteria in wastewater treatment
Year
2024
Publication Outlet
Journal of Water Process Engineering, Vol. 65, 105772
DOI
ISBN
ISSN
2214-7144
Citation
Al-Ani, Sama; Guo, Hui; Fyfe, Sheila; Long, Zebo; Donnaz, Sylvain; Kim, Younggy (2024) Deep learning-based image analysis for filamentous and floc-forming bacteria in wastewater treatment, Journal of Water Process Engineering, Vol. 65, 105772,
https://doi.org/10.1016/j.jwpe.2024.105772
Abstract
In municipal wastewater treatment, effective secondary clarification relies on the balance between floc-forming bacteria and filamentous bacteria. Consequently, comprehensive and real-time monitoring of this balance will enable reliable operation of biological wastewater treatment. This research presents an artificial intelligence (AI)-based approach for the classification of filamentous and floc-forming bacteria in microscopic images using deep learning. To provide ground truth labeling, an automated rule-based segmentation algorithm was developed using color and morphology criteria along with supplementary filtration steps to enhance the precision of filamentous and floc-forming bacteria identification. The segmentation algorithm demonstrated reliable detection and categorization of bacteria across varying background intensities and effectively recognized intricate microbial configurations. Subsequently, the supervised deep learning model was trained on the segmented images and constructed with an encoder/decoder architecture. Machine training with only 68 microscopic images demonstrated successful classification of the filamentous and floc-forming bacteria with a 97.8 % accuracy. In addition, qualitative evaluation demonstrated that the deep learning model could generalize machine understanding across diverse scenarios and discern misclassified filamentous bacteria accurately. The proposed model stands as a promising automated tool for real-time quantification of filamentous and floc-forming bacteria in bioreactors and clarifiers, offering the potential for reliable operation as well as immediate actions for sludge bulking and membrane fouling problems.
Plain Language Summary
Section 2: Additional Information
Program Affiliations
Project Affiliations
Submitters
Publication Stage
Published
Theme
Presentation Format
Additional Information
Keywords: Deep learning; Sludge bulking; Image segmentation; Filamentous bacteria; Floc-forming bacteria