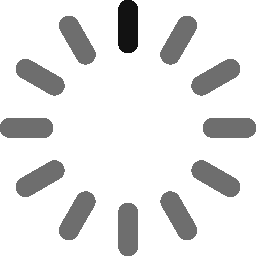
Related items loading ...
Section 1: Publication
Publication Type
Journal Article
Authorship
Vidrio-Sahagún, Cuauhtémoc Tonatiuh; He, Jianxun; Pietroniro, Alain
Title
Improved Correction of Extreme Precipitation Through Explicit and Continuous Nonstationarity Treatment and the Metastatistical Approach
Year
2025
Publication Outlet
John Wiley & Sons, Ltd, Water Resources Research, Vol. 61, Iss. 1, e2024WR037721
DOI
ISBN
ISSN
0043-1397
Citation
Vidrio-Sahagún, Cuauhtémoc Tonatiuh; He, Jianxun; Pietroniro, Alain (2025) Improved Correction of Extreme Precipitation Through Explicit and Continuous Nonstationarity Treatment and the Metastatistical Approach, John Wiley & Sons, Ltd, Water Resources Research, Vol. 61, Iss. 1, e2024WR037721,
https://doi.org/10.1029/2024WR037721
Abstract
Climate models simulate extreme precipitation under nonstationarity due to continuous climate change. However, systematic errors in local-scale climate projections are often corrected using stationary or quasi-stationary methods without explicit and continuous nonstationarity treatment, like quantile mapping (QM), detrended QM, and quantile delta mapping. To bridge this gap, we introduce nonstationary QM (NS-QM) and its simplified version for consistent nonstationarity patterns (CNS-QM). Besides, correction approaches for extremes often rely on limited extreme-event records. To leverage ordinary-event information while focusing on extremes, we propose integrating the simplified Metastatistical extreme value (SMEV) distribution into NS-QM and CNS-QM (NS-QM-SMEV and CNS-QM-SMEV). We demonstrate the superiority of NS- and CNS-QM-SMEV over existing methods through a simulation study and show several real-world applications using high-resolution-regional and coarse-resolution-global climate models. NS-QM and CNS-QM reflect nonstationarity more realistically but may encounter challenges due to data limitations like estimation errors and uncertainty, particularly for the most extreme events. These issues, shared by existing approaches, are effectively mitigated using the SMEV distribution. NS- and CNS-QM-SMEV offer lower estimation error, approximate unbiasedness, reduced uncertainty, and improved representation of the entire distribution, especially for samples of ~70 years, and greater superiority with larger samples. We show existing methods may perform competitively for short samples but exhibit substantial biases in quantile-quantile matching due to bypassing nonstationarity modeling. NS- and CNS-QM-SMEV avoid these biases, adhering better to their theoretical functioning. Thus, NS- and CNS-QM-SMEV enhance the correction of extremes under nonstationarity. Yet, properly identifying nonstationarity patterns is crucial for reliable implementations.
Plain Language Summary
Section 2: Additional Information
Program Affiliations
Project Affiliations
Submitters
Publication Stage
Published
Theme
Presentation Format
Additional Information
Keywords: nonstationary bias correction; simplified metastatistical extreme value distribution; quantile-quantile matching; ordinary-event data; quantile-wise and distribution-wise assessment