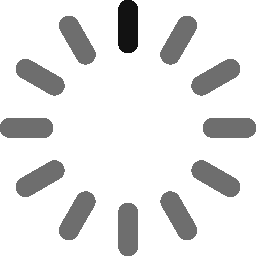
Related items loading ...
Section 1: Publication
Publication Type
Journal Article
Authorship
Chang, Q., Zwieback, S., Berg, A.
Title
The Predictive Skill of a Remote Sensing-Based Machine Learning Model for Ice Wedge and Visible Ground Ice Identification in Western Arctic Canada
Year
2025
Publication Outlet
Remote Sens. 2025, 17(7), 1245
DOI
ISBN
ISSN
Citation
Chang, Q., Zwieback, S., Berg, A. (2025). The Predictive Skill of a Remote Sensing-Based Machine Learning Model for Ice Wedge and Visible Ground Ice Identification in Western Arctic Canada. Remote Sens. 2025, 17(7), 1245
https://doi.org/10.3390/rs17071245
Abstract
Fine-scale maps of ground ice and related surface features are critical for permafrost-related modelling and management. However, such maps are lacking across almost the entire Arctic. Machine learning provides the potential to automate regional fine-scale ground ice mapping using remote sensing and topographic data. Here, we evaluate the predictive skill of XGBoost models for identifying (1) ice wedge and (2) top-5m visible ground ice in the Tuktoyaktuk Coastlands. We find high predictive skill for ice wedge occurrence (ROC AUC = 0.95, macro F1 = 0.80), with the most important predictors being slope, distance to the coast, and probability of depression. The model accurately predicted regional and local trends in ice wedge occurrence, with an increase in ice wedge polygon (IWP) probability towards the coast and in poorly drained depressions. The model also captured IWP in well-drained uplands of the study area, including locations with poorly visible troughs not contained in the training data. Spatial transferability analyses highlight the regional variability of ice wedge probability, reflecting contrasting climatic and surface conditions. Conversely, the low predictive skill for visible ground ice (ROC AUC = 0.67, macro F1 = 0.53) is attributed to limitations in training data and weak associations with the remotely sensed predictors. The varying predictive accuracy highlights the importance of high-quality reference data and site-specific conditions for improving ground ice studies with data-driven modelling from remote sensing observations.
Keywords: ice wedge; remote sensing; machine learning; ground ice
Plain Language Summary